
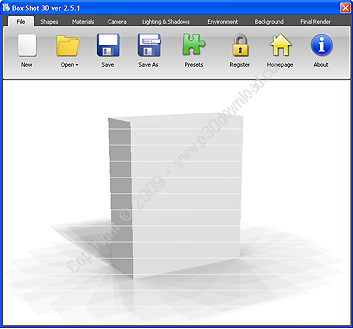
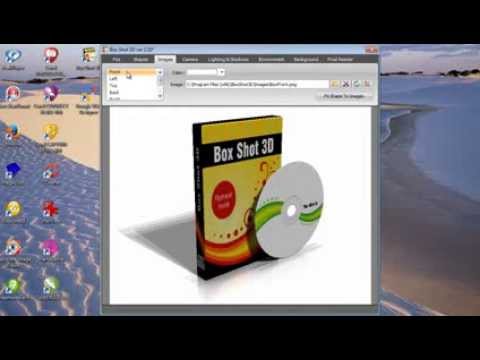
We acknowledge the significant efforts and suggestions of the reviewers. We also thank Elys Muda for allowing use of the video. We thank David Luebke, Jan Kautz, Peter Shirley, Alex Evans, Towaki Takikawa, Ekta Prashnani and Aaron Lefohn for feedback on drafts and early discussions. Without ViT layers, our method cannot capture the details of the subject, but retains the ability to predict a coherent 3D representation. We provide videos to demonstrate the importance of these design decisions. We use strictly synthetic data to train our model, but find that generalization in-the-wild requires sampling geometric camera parameters from distributions (instead of keeping them constant as in EG3D) in order to better align the synthetic data to the real world. We found the architecture of the encoder to be integral to the performance of our model and propose to incorporate Vision-Transformer (ViT) layers to allow the model to learn highly-variable and complex correspondences between 2D pixels and the 3D representation. Our method directly predicts a canonical triplane representation for volume rendering conditioned on an unposed RGB image. Our algorithm can also be applied in the future to other categories with a 3D-aware image generator.įor business inquiries, please visit our website and submit the form: NVIDIA Research Licensing.įigure 1: Overview of our encoder architecture and training pipeline for our proposed method. We showcase our results on portraits of faces (FFHQ) and cats (AFHQ), but The state-of-the-art methods, demonstrating significant improvements in robustness and image quality inĬhallenging real-world settings. Technical contributions include a Vision Transformer-based triplane encoder, a camera dataĪugmentation strategy, and a well-designed loss function for synthetic data training.
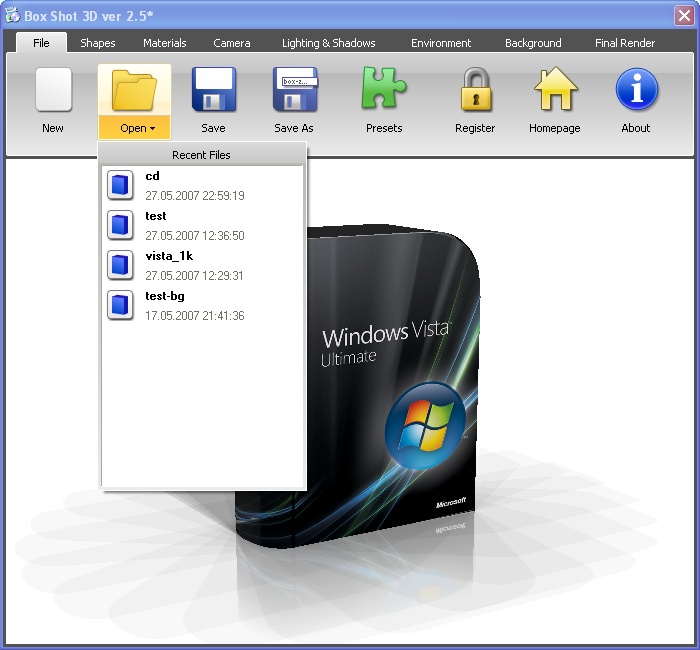
BOX SHOT 3D IMAGES HOW TO
Only synthetic data, showing how to distill the knowledge from a pretrained 3D GAN into a feedforwardĮncoder. To train our triplane encoder pipeline, we use GAN-inversion baselines that require test-time optimization. Our method is fast (24 fps) on consumer hardware, and produces higher quality results than strong Given a single RGB input, our image encoder directly predicts aĬanonical triplane representation of a neural radiance field for 3D-aware novel view synthesis via volume Image (e.g., face portrait) in real-time. We present a one-shot method to infer and render a photorealistic 3D representation from a single unposed
